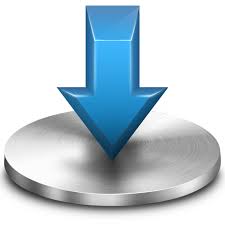
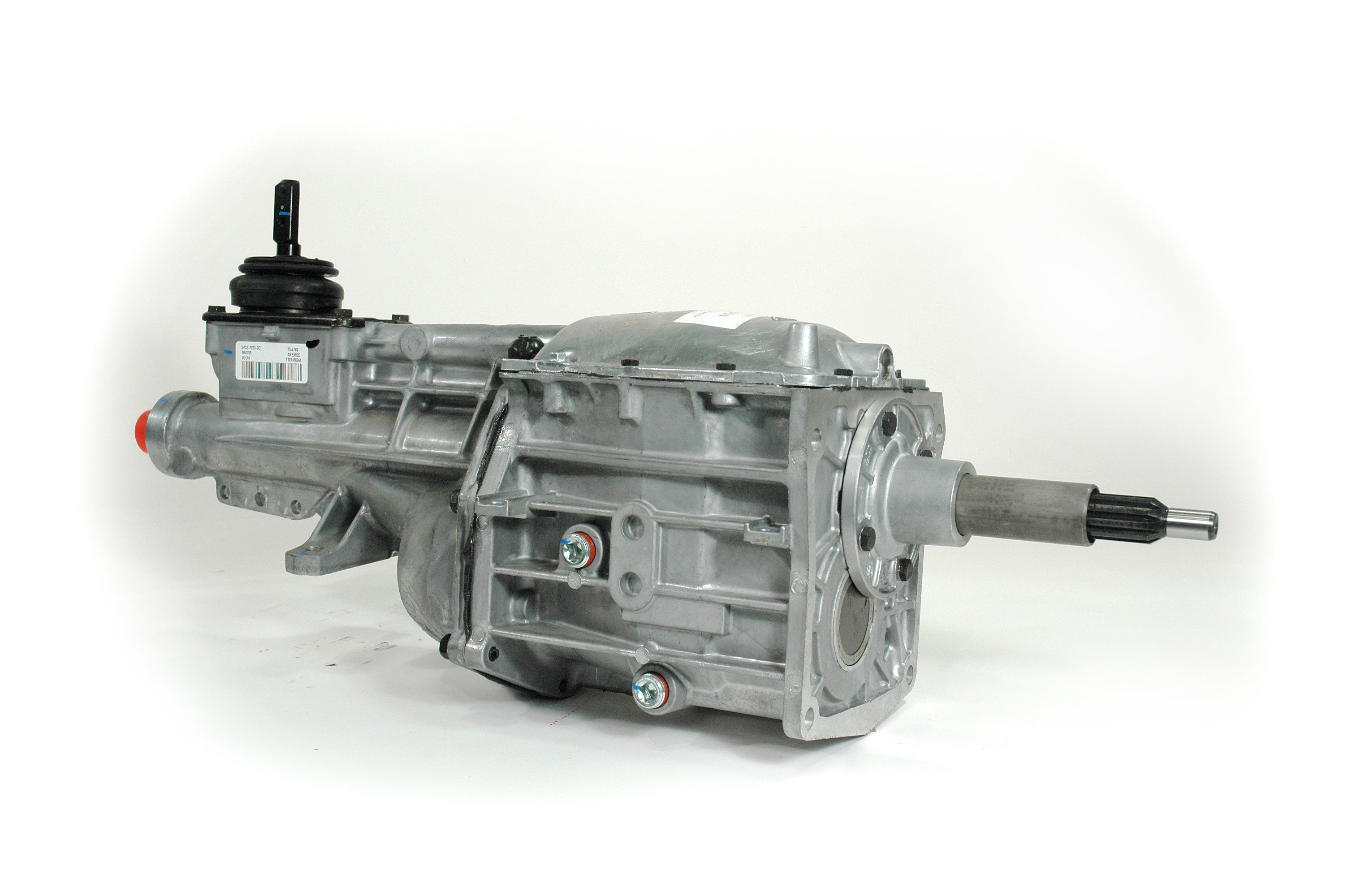
The final option is pertaining only on supervised tasks and fine-tuning on the same set of supervised tasks, which quite similar to what computer vision does. The result shows that this strategy still generates pretty good pretrained model. Leave-one-out multi-tasks training is pertaining on a multi-task mixture and then fine-tuning on a task which isn't used during pertaining. This strategy closes the gap between unsupervised pertaining and fine-tuning. Another option is offered by MT-DNN : training on a multitask mixture and then fine-tuning on each individual task. Original one is pretraining on unsupervised tasks and fine-tuning on each individual downstream tasks. source: Source: Collin Raffel videoīesides multi-task training, there are also other training strategies. Especially on GLUR and SQUAD and superGLUE, there is a significant drop in performance when trying to train a single model on all these tasks at once.ĭifferent training strategies and their performance. Setting threshold and temperatures correctly would have somewhat similar performance to the pretrained and fine-tuned setting. Training equally on multi-tasks leads to worse performance. We can also take these number of example proportions and apply a temperature to them to make then more close to uniform. This is what multilingual BERT does - sampling from different languages. Because the unsupervised task is so big so that we need to set an artificial limit on how much we train on that task. The next option is to weight each dataset by the number of examples in the dataset as we don’t want to overfit the small dataset and underfit the big dataset. Instead of doing unsupervised pretraining and fine-tuning, the model is trained on multi-tasks. Different mixing strategies and their performance.
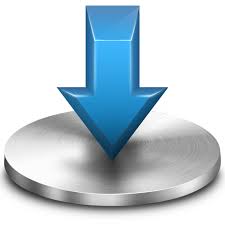